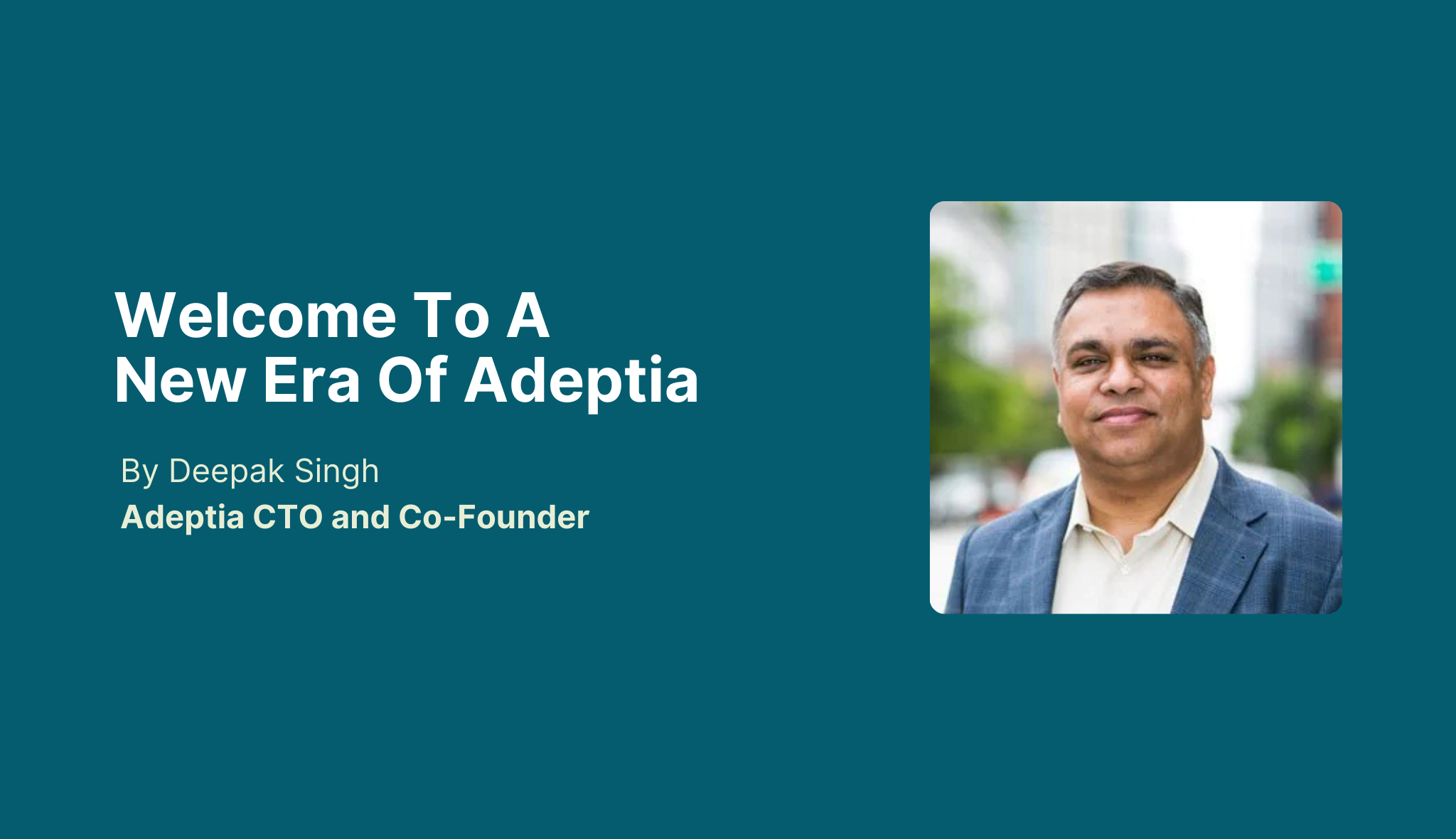
Welcome to a New Era of Adeptia Connect
Today, Adeptia launched the latest version of Adeptia Connect, which includes several exciting new enhancements! Hear from CTO and cofounder Deepak Singh what this means for our customers and prospects.
Cutting-edge AI automatically extracts insights from documents like purchase orders, invoices, and loan applications, and transforms it into your preferred format for integration with your systems.
Manual data entry is slow and error-prone. Adeptia’s IDP solution is 99%+ accurate, so you can spend less time on data review.
Shrink document processing time from days to minutes. Process documents as soon as they’re received and automatically integrate the data into existing workflows.
Free Trial
Resources
Adeptia’s Intelligent Document Processing (IDP) solution transforms the way businesses handle and analyze data by automating the extraction and conversion of unstructured and semi-structured informati...
This whitepaper provides an in-depth exploration into the integration of Generative AI (GenAI) with Retrieval Augmented Generation (RAG) technologies in the business domain, offering substantial insig...
Self-service integration technology is enabling organizations elevate levels of productivity by empowering all business users to harness the true potential of data with minimum reliance on IT....